Histopathology refers to the analysis of histological sections taken from a biopsy or other tissue by a pathologist. In the clinical setting, histopathology is traditionally used to analyze tissues for diseases such as evaluating a breast tumor biopsy for cancer or a sentinel lymph node for metastasis. Information such as stage and class can be determined through this process and the appropriate treatment regimen for a patient can be devised in connection with other interrogation techniques. In the research setting, histopathology is used for a wide range of applications from evaluating the toxicity of a new compound to the spread of a virus in an animal model. Histopathology is foundational to most life science fields as it is our principle tool for evaluating the microscopic features of tissues and diagnosing many diseases. However, the current histopathology paradigm has two major limitations and areas for improvement which companies and research groups are working to improve:
Histological Sampling Bias
The practice of histopathology relies upon the qualitative analysis of ultra-thin two-dimensional slices that have been removed from three-dimensional biopsies. The inherent problem with using two-dimensional slices to characterize a biopsy is that this practice makes the assumption that the biopsy is relatively homogensis and that information gathered from one slice can be extrapolated to the whole biopsy. This sampling bias is further worsened because the slices that are analyzed are a subset of the data within the biopsy and the biopsy is a subset of the data within the tumor. It is possible that if the biopsy is not appropriately targeted that only a small portion of the tumor will be excised which means that an important feature could be missed if the appropriate section is not taken. This sub sampling problem is intensified as heterogeneity in a tissue increases and thus can lead to inaccurate conclusions about the characteristics of a particular disease.
In the research space, researchers have begun to approach this problem through the adoption of 3D histology where tissues are either sectioned into hundreds to thousands of slices (e.g. serial sectioning) which are later pieced back together with software or through the use of tissue clearing and 3D microscopy (e.g. confocal, light sheet, two-photon). While both techniques have shown promise in the research space, the current histopathology paradigm has significant inertia and it will take overwhelming peer-reviewed support to introduce a new dimension to histopathology analysis. It should also be stressed that such three-dimensional microscopy techniques are only beneficial for highly complex and heterogeneous tissues whereas traditional 2D histology is adequate in most cases.
Human-based Qualitative Analysis
The second major problem with the current histopathology paradigm is that it relies upon humans to interpret two-dimensional slides and to render pathology reports based upon what they see. It has been shown that there is substantial inter and intra pathologist variability in how they analyze slides and that the process is highly subjective. This limitation combined with sampling bias can lead to pathologist’s incorrectly characterizing pathology slides.
The interesting thing about this limitation is that we now have the ability and technology to greatly improve slide characterization through the use of advances in computing power and machine learning. Computers by their nature are incredibly good at analyzing imaging data and extracting thousands of features which can be quantitatively analyzed. Researchers have shown that computer vision algorithms improve diagnosis accuracy for many diseases when compared to human pathology experts. These algorithms can run 24/7 without getting tired and can transform histopathology from a qualitative art to a quantitative science. Additionally, these algorithms can analyze thousands of features that a human could never interpret and compare them to a library of millions of known samples in less than a second.
However, one of the issues with this digital pathology approach is that even though it has been shown to improve diagnosis accuracy by several groups including Google, PathAI and Philips, it will take a long period of time for it to be adopted widely as the histopathology field is well entrenched in its current qualitative paradigm. Additionally, the use of more accurate algorithms would in the long-term replace the need for many highly trained pathologists.
At Visikol, we are addressing these limitations through our 3Sscreen services which combine high-throughput 3D tissue imaging with quantitative image analysis and custom digital pathology solutions for our clients. We offer full-service digital pathology solutions from slide scanning all the way to the development of customized algorithims and software applications.
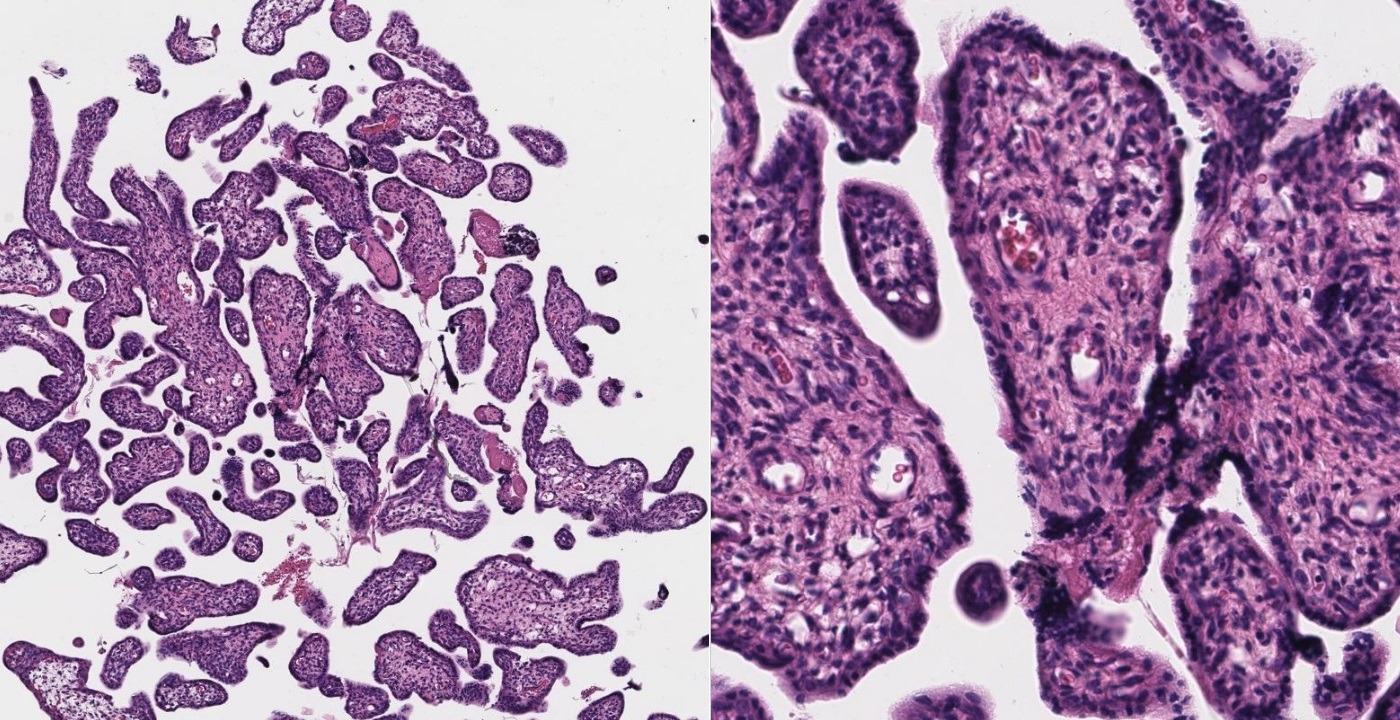
H&E of Human Placenta Tissue