Visikol generates and analyzes hundreds of images per week in order to extract quantitative endpoints to help answer clients’ research questions. Visikol uses various method of analysis, and one of the many programs used is ImageJ, where the macro language is utilized to run batch analysis. However, when generating a plethora of images per week, there are bound to be some images that are less than ideal for analysis and cannot be re-imaged. These images may be slightly out of focus, have artifacts, have high background signal, or a less than ideal intensity histogram. Therefore, to tackle these issues, there are several steps that can be taken to work around these hindrances.
The Simple Solution:
The simplest solution would be to annotate around regions with artifacts, high background signal, or blurriness. Although, this solution would only work if there were regions in the images that are of good quality for analysis, and it would be a tedious solution if you need to analyze many images with similar issues.
Dealing with Blur:
For out of focus images, utilizing a deconvolution algorithm is a great way to alleviate the blurriness and sharpen whatever is being looked at. Deconvolution is a common image processing technique that is utilized to improve the contrast and resolution of images by deblurring images.
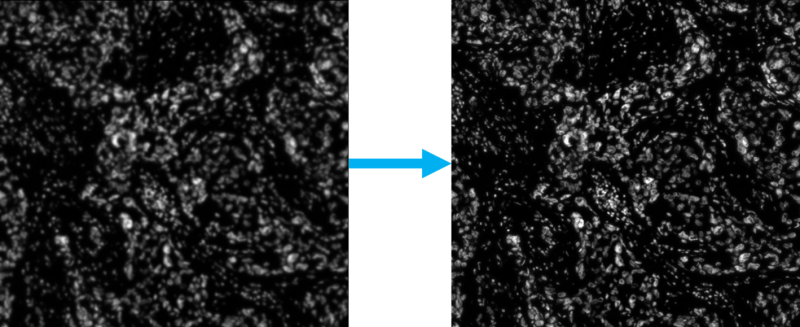
Figure 1. An example of deconvolution being applied to an image.
Handling Artifacts and High Background Signal:
When dealing with artifacts and high background signal, there is no simple solution other than annotating around them. However, this may not be a feasible task when asked to analyze a large magnitude of images, which is why it becomes important to minimize the pickup of this noise in your analysis. This can be done through several different methods and depends on the type of analysis being performed. If you are trying to segment out a particular object that has a specific shape, then you can avoid noise by filtering the objects by size and shape to get rid of objects that do not match the general shape of what you are interested in. Additionally, when analyzing intensity of images, a good way to avoid high background signal or artifacts is finding out the intensity range in which the features of interest are in the intensity histogram and removing all signal above or below that range in the analysis. Click here for more on Noise Reduction Methods in ImageJ.
Adjusting Poor Intensity Histograms:
With poor intensity histograms, it is important to be able to separate the background and foreground signal. Therefore, a contrast adjustment or histogram equalization algorithm is useful in improving the signal in the image. This works by stretching or trying to flatten out the intensity histogram so that there is a better distribution of intensity values along the full intensity range.
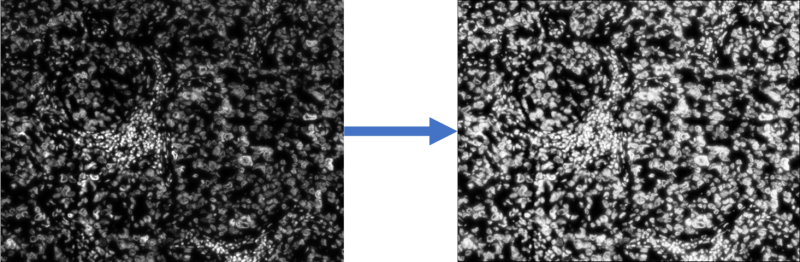
Figure 2. An example of a local contrast adjustment algorithm applied to an image.
When dealing with poor quality images, there are many ways to improve or avoid errors in imaging such as those listed above. At Visikol, we are experts at handling poor quality due to the shear volume of images we deal with on a day-to-day basis. Please feel free to contact us for your Image Analysis needs.